Introduction
Data reconciliation refers to comparing data from different sources to ensure consistency, accuracy, and timeliness. It’s a critical process for establishing and maintaining a “single source of truth” – a fundamental goal of enterprise data projects. Think of it like double-checking the numbers before you file a report—except on a larger scale.
It could involve comparing sales data across multiple platforms, verifying that customer information is correct across departments, or ensuring that transactions match up with records in your accounting software. Without proper reconciliation, organizations often struggle with conflicting data versions, leading to poor decision-making and reduced operational efficiency.
By regularly reconciling data, businesses can avoid discrepancies, reduce errors, and maintain the overall integrity of their data.
💡 Use accurate location data for data reconciliation. Our worldwide zip code and address database is updated weekly, relying on over 1,500 sources. Browse GeoPostcodes datasets and download a free sample here.
The Importance of Data Reconciliation
So, why is data reconciliation so crucial? Inconsistent or flawed data can lead to:
- Poor decision-making: Basing decisions on inaccurate data can lead to costly mistakes.
- Compliance issues: Many industries, like finance and healthcare, require accurate data for regulatory compliance.
- Operational inefficiencies: When data doesn’t align across systems, more time is spent fixing errors, which reduces productivity.
- Customer dissatisfaction: Data inaccuracies can cause issues like sending incorrect bills, shipping the wrong products, or delivering poor customer service.
By reconciling data, businesses ensure they work with the most accurate information possible, enhancing trust and operational efficiency.
The Data Reconciliation Process
Data reconciliation processes vary depending on the industry and the complexity of handling data, but most follow a similar framework. Here’s a step-by-step look at how data reconciliation typically unfolds:
1. Data Extraction
First, you need to extract data from different sources. This might include pulling information from a CRM, accounting software, spreadsheets, or external data providers. The goal is to compare all relevant data in one place.
2. Data Transformation
Once the data is collected, it must be transformed into a consistent format. This could mean standardizing dates, currency formats, or customer names across different systems. Data transformation is crucial for ensuring data comparability.
3. Data Validation
Before diving into reconciliation, it’s essential to validate the data. Data validation checks ensure that the information meets the required standards—whether it’s verifying its fields are complete, data formats are correct, or entries match predefined rules. This step is critical for catching errors early in the process.
4. Data Comparison
Now comes the core of the reconciliation process: data comparison. This involves matching data from different sources and identifying discrepancies. For example, you might compare financial transactions from two accounting systems or sales records from multiple retail locations.
5. Error Resolution
When data inconsistencies are identified, they need to be addressed. This step involves investigating and resolving the discrepancies manually or through automated processes. Sometimes, this might mean updating records, removing duplicate entries, or correcting errors at the source.
6. Reporting and Documentation
Finally, generating reports documenting the reconciliation process is essential after discrepancies are resolved. These reports record what discrepancies were found and how they were resolved and ensure that the data is now aligned.
Automated Data Reconciliation: The Smart Approach
As data volumes grow, manually reconciling data can become overwhelming and error-prone. Enter automated data reconciliation—a game changer for businesses managing large datasets. Benefits of automated data reconciliation:
- Speed: Automated systems can reconcile vast amounts of data in seconds, saving time and reducing the need for human intervention.
- Accuracy: Automated systems minimize human error by reducing the reliance on manual processes, ensuring more reliable results.
- Real-time Updates: Automated tools can provide real-time reconciliation, helping businesses catch and resolve discrepancies as they arise.
- Scalability: As your business grows and handles more data, automated reconciliation can scale with you, keeping up with increasing data demands.
Businesses can streamline the process by incorporating data reconciliation software, ensuring data is consistently aligned across platforms.
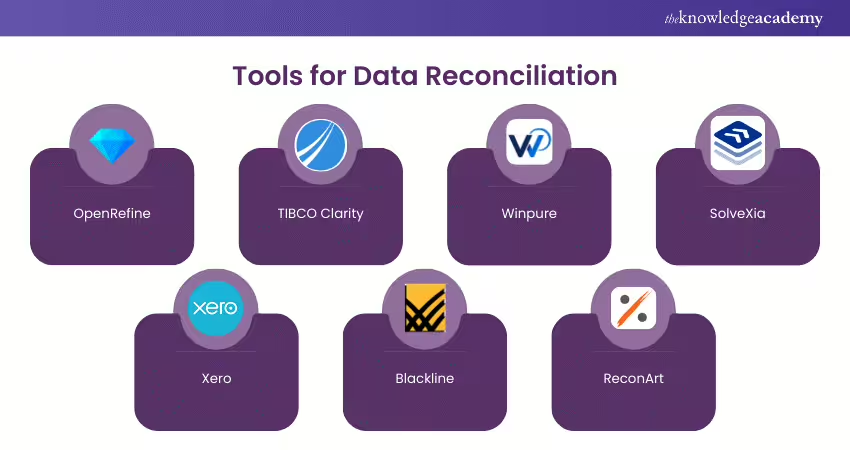
Data Reconciliation Tools: What to Look For
Choosing the correct data reconciliation tool is essential for any organization looking to improve the accuracy and efficiency of its data management processes. Here’s what to Here’sor in the right tool:
- Advanced-Data Matching Algorithms: Ensure your tool has robust algorithms capable of comparing large datasets and accurately identifying discrepancies.
- Exception Management: Good reconciliation tools will identify errors and offer solutions to resolve them quickly.
- Real-time Integration: The ability to integrate with your existing systems in real-time ensures that your data is always current and up to date.
- User-friendly Reporting: Your tool should provide clear, detailed reports documenting the reconciliation process, making tracking and resolving issues easy.
- Secure Data Transfer: Ensure the tool offers secure mechanisms for transferring and comparing data to avoid breaches or data loss during reconciliation.
Data Quality: The Foundation of Reconciliation
Ensure data quality before you start reconciling data. High-quality data is accurate, complete, and consistent—the foundation of any successful reconciliation process. Without data quality checks, flawed or inaccurate data can undermine the reconciliation process.
- Regular Data Audits: Performing regular audits helps catch issues early and ensures your data stays accurate.
- Standardization: Ensure all data follows the same format and standards to make reconciliation easier.
- Data Validation: Check that data inputs meet the required criteria and flag any inconsistencies early in the process.
Data Integrity and Consistency: The Goal of Reconciliation
The ultimate goal of data reconciliation is to maintain data integrity and consistency. When data is accurate, complete, and consistent, it builds trust across departments, helps avoid operational inefficiencies, and supports better decision-making.
Maintaining data integrity involves ensuring that data remains accurate and consistent over time, even as it moves across systems and transforms. Regular reconciliation processes, data validation, and quality checks help maintain integrity across systems.
Data Migration and Reconciliation: A Common Challenge
Data migration presents a significant challenge whenever organizations move data from one system to another—whether during system upgrades, mergers, or integrations. Ensuring that data remains consistent before, during, and after migration is critical, and data reconciliation plays a vital role in this process.
Steps to Reconcile Data During Migration:
- Pre-migration Reconciliation: Ensure that data is accurate and complete before migration begins.
- Post-migration Validation: After the migration, validate that data in the new system matches the original records.
- Ongoing Reconciliation: Set up ongoing reconciliation to ensure that any discrepancies introduced during the migration process are caught and resolved.
💡 Use accurate location data for data reconciliation. Our worldwide zip code and address database is updated weekly, relying on over 1,500 sources. Browse GeoPostcodes datasets and download a free sample here.
Data Reconciliation Techniques and Methods
There are various data reconciliation methods organizations can use, depending on the complexity of the data and the specific requirements:
- Master Data Reconciliation: Focuses on aligning key datasets, like customer records, across systems.
- Transactional Data Reconciliation: Ensures that individual transactions, such as sales or payments, are consistent across systems.
- Automated Reconciliation: Uses reconciliation software to handle large, complex datasets in real-time.
- Manual reconciliation is often used for smaller datasets or when automated tools aren’t available, though it can be time-consuming and error-prone.
Best Practices for Effective Data Reconciliation
Implementing a practical data reconciliation framework involves more than having the right tools. Here are some best practices:
- Automate When Possible: Automation saves time and reduces errors.
- Reconcile Frequently: Regular reconciliation helps catch issues early before they become bigger problems.
- Monitor Data Quality: Perform ongoing data quality checks to ensure your data stays clean.
- Document Your Processes: Clear documentation of the reconciliation process helps standardize operations and ensures accountability.
Conclusion
At the core of every thriving business is solid, reliable data. Whether you’re looking to streamline operations, meet regulatory standards, or provide top-notch customer service, data reconciliation is critical to ensuring the data you’re working with is accurate and dependable.
Using the right processes, tools, and best practices outlined here, companies can keep their data clean, avoid costly errors, and boost overall performance.
GeoPostcodes makes reconciling location-based data across various systems easy, ensuring information like addresses and postal codes is always up-to-date and accurate. You can check out the GeoPostcodes database for free and download a free sample here.
FAQ
What is an example of data reconciliation?
An example of data reconciliation is comparing a customer list sent from a third party with your internal list of customers to identify and resolve discrepancies, such as duplicate entries or incorrect contact details.
Why is data reconciliation required?
Data reconciliation is required to ensure data consistency, accuracy, and completeness across multiple sources or systems.
It helps detect anomalies, maintain compliance with regulations, resolve discrepancies, and improve overall data quality.
It is essential for accurate reporting, decision-making, and preventing errors in various business operations, including financial and cybersecurity contexts.
What is the difference between data validation and data reconciliation?
Data validation involves checking data against predefined rules and standards to ensure accuracy, completeness, and consistency before or during the data processing stage.
On the other hand, data reconciliation is comparing and aligning data from different sources or systems to identify and resolve discrepancies after the data has been transferred or processed.
What are the best practices for data reconciliation?
Best practices for data reconciliation include:
- Automate the process using automated reconciliation software to reduce manual errors and increase efficiency.
- Standardize data formats and terminologies to ensure consistency across different systems.
- Perform regular reconciliations to identify and rectify discrepancies promptly.
- Implement strict data validation checks to ensure data accuracy and integrity.
- Conduct regular audits to identify and rectify discrepancies.
- Establish a defined process with clear steps for data collection, comparison, discrepancy resolution, and reporting.
- Use data integration tools to map different data schemas to a common model.
- Monitor key performance indicators to assess the effectiveness of the reconciliation process.
- Continuously improve the process based on feedback and evolving business requirements.
Why is data reconciliation important for businesses?
Data reconciliation is crucial for ensuring data across different systems is consistent and accurate.
Without proper reconciliation, businesses risk making decisions based on inaccurate or incomplete data, leading to operational inefficiencies.
Regular data reconciliation helps identify and resolve discrepancies, improving data reliability and boosting overall business performance.
How can inconsistent data affect business operations?
Inconsistent data can cause significant problems in business operations, such as incorrect reporting, poor decision-making, and inefficiencies in processes.
For instance, mismatched data across systems can lead to customer information, order management, and financial records errors.
Addressing data consistency ensures smoother workflows and better data-driven decisions, ultimately enhancing operational efficiency.